I conducted this research with a team under Bass Connections at Duke University.
Cost-effectiveness analysis is a type of economic analysis that is used to compare the effectiveness of different intervention strategies. The effectiveness can be measured using different metrics like Quality Adjusted Life Years (QALY), Disability Adjust Life Years (DALYs), Incremental Cost Effectiveness Ratio (ICER), etc.
Public health interventions require money and public institutions don’t have unlimited resources to invest. They are trying to find an optimum strategy that will help them decide which intervention strategy would help improve lives and justify the cost.
For our project, we mainly focussed on intervention strategies for different Non-Communicable Diseases (NCDs) like heart attacks, cancers, diabetes, etc. which are occurring more frequently than communicable diseases across the globe, including developing countries.
On the technical side, we made use of a dynamic modeling system – Stochastic Rewards Net, which helps us model a population/control group and model their lifecycle with and without intervention strategies. We create different health states related to the disease and make use of probabilities and statistical distribution. In its simplicity, it’s similar to Markov Chains but with more features and modalities. It makes use of different transition states, has a time component added to the transitions, and helps to add conditional movement of individuals in a population from one state to another.
We did a literature review of several papers that performed CEA based on Markov Chains, understood their work, and imitated the same in SRN using a tool built by one of our mentors, Prof. Kishore Trivedi. We also made modifications to our adaptation based on input provided by Public Health Professor to make everything epidemiologically consistent.
The result is a Systemization of Knowledge (SoK), a paper we are currently working on as a team.
Our team presented a poster of our work at the Bass Connections showcase.
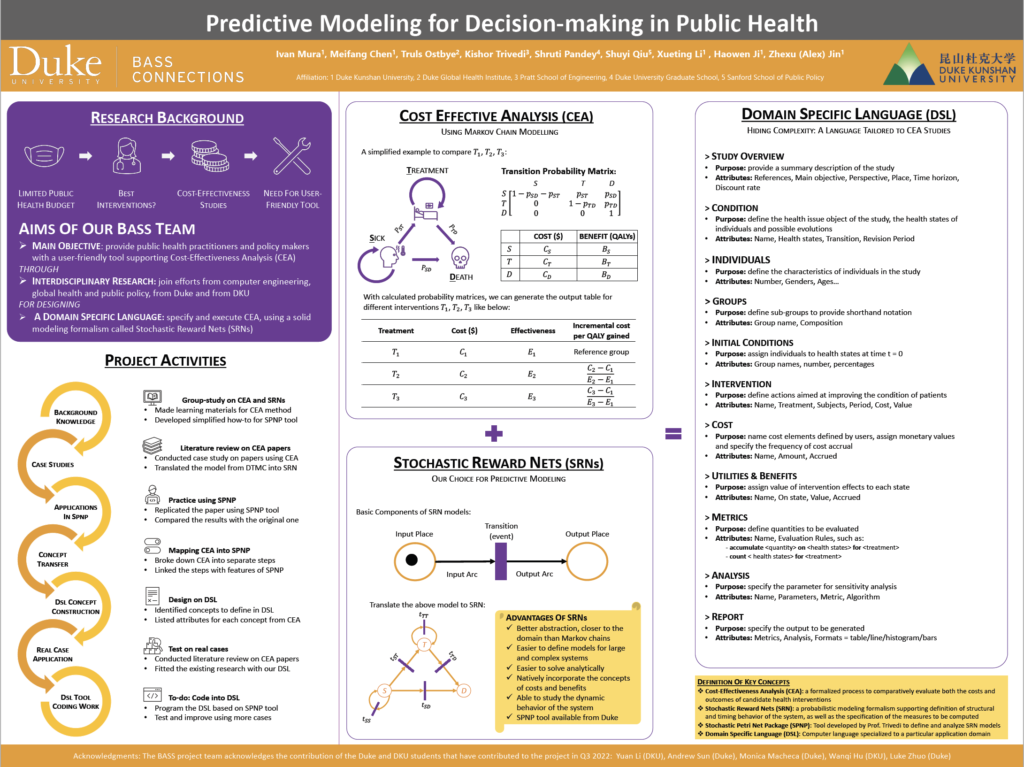
Other team members: Shuyi, Alex, Haowen, and Xeuting.